With a slight delay, I've realised that the excitement surrounding Generative AI is more than just hype. So, I finally decided to learn more about it.
Recently, Andrew Ng — an AI expert renowned for making AI education accessible through online courses — released the course "Generative AI for Everyone" on Coursera.
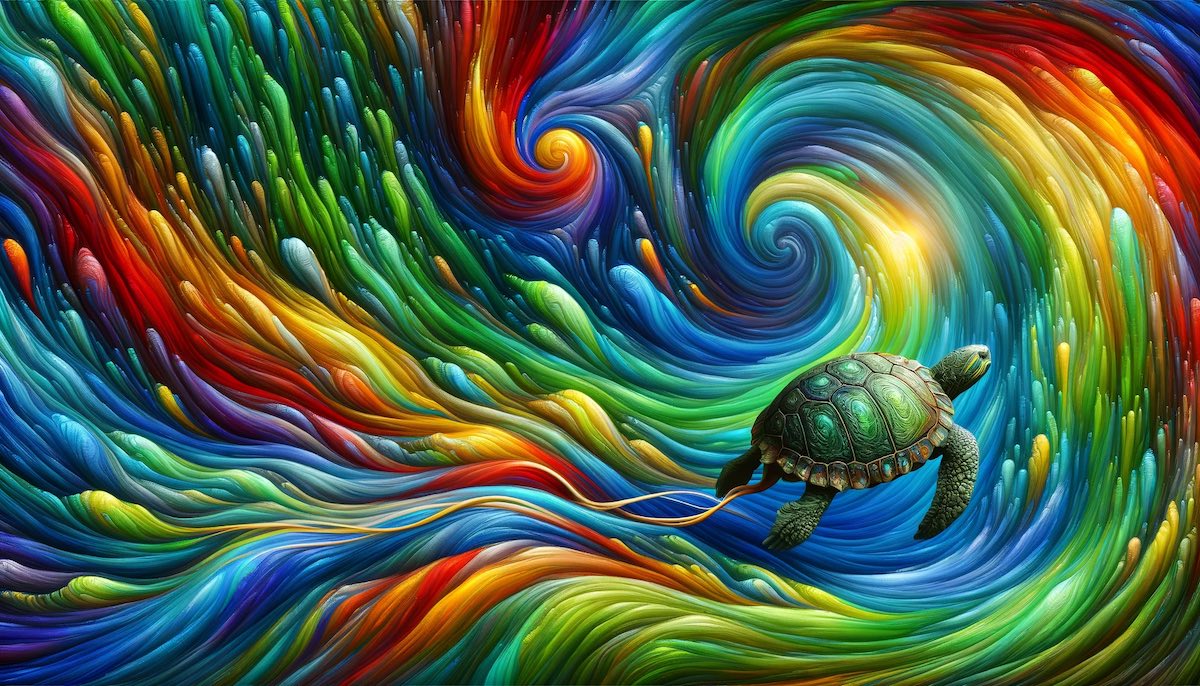
Image by Will Fish
I took the course, which was worth the time and the money (40£).
It is an introductory course, perfect for anyone who is not an expert in Machine Learning (ML), like myself.
Andrew talks about Large Language Models (LLM) and techniques like Retrieval Augmented Generation (RAG) and fine-tuning. He explains all the Generative AI concepts in simple terms without going into detail, making the course accessible.
The course is divided into three weeks.
In the first week, he explains what Generative AI can and can't do. In short, it can write text for you, proofread a document, summarize, or extract information from text. It can also create interactive chatbots.
In the second week, Andrew goes deeper than just LLM prompting. He introduces how to specialize an LLM using Retrieval Augmented Generation (RAG) and fine-tuning.
In the third week, he talked about the impact of Generative AI on society. He discussed people's fears and the ethical implications of AI in general.
I found this last week very interesting, and I want to share some of my notes:
Fear of Job Loss:
People fear AI can cause the loss of numerous jobs. However, any job comprises various tasks, and AI can only help in some tasks. As a software developer, I had the same fear after seeing how easily Copilot or ChatGPT can generate code.
But those systems will not replace developers, mainly for two reasons: The suggested code is often unsuitable for the issue the developer is working on, even if it is a good starting point. Writing code is one of the numerous tasks developers perform (like talking to product designers and stakeholders, estimating tasks, pairing and training other developers, ...)
Andrew cites the example of the radiologist, where AI cannot replace a radiologist due to the complexity of her tasks, but it helps a lot to interpret the image scans. In other words, AI can augment professions rather than replace them.
Fear of AI Amplifying Humanity's Worst Impulses:
LLMs are trained on text from the Internet, which sometimes contains bias, hatred, and misconceptions. These negative qualities might be present in the output generated by LLM chat. AI researchers are making excellent progress in reducing them in Language Models. They successfully use techniques like fine-tuning and reinforcement learning from human feedback (RLHF) to minimize biases.
Fear of Human Extinction:
Arguments on human extinction often need to be more specific and clear. New technologies can initially have side effects that are difficult to predict, but humanity has historically managed powerful technologies responsibly.
For example, when electrification was introduced, people feared it. It was not safe, and some people died or were injured by electrocution. Now, electricity in our homes is secure, and nobody is afraid to turn the light on.
In the same way, poorly designed self-driving cars led to accidents. A lawyer cited fake cases generated by LLM in a legal brief. Other AI systems caused issues or unexpected side effects. We must learn to build control measures to ensure AI's safe development.
Anyway, these accidents are very far from causing human extinction.
Andrew did not address the use of AI for military purposes. In this case, AI systems are deployed on purpose to cause harm to humans. Imagine the harm thousands of autonomous drones or armed robot dogs can do if deployed in a city. It may be comparable to the atomic bombs.
On the other hand, AI is essential for addressing challenges like climate change. AI is a tool to increase humanity's chances of thriving over the next thousand years.
Responsible AI:
Governments and companies are working on frameworks for responsible AI systems. Some of the key points of those frameworks are: Fairness: ensure AI doesn't perpetuate biases. Transparency: make AI systems and decisions understandable. Privacy: protect user data and assure confidentiality. Security: safeguard AI systems from malicious attacks. Ethical use: ensure AI is used for beneficial purposes. Defining a framework is complex due to the ambiguity of ethical decisions.
Conclusion
Delving into Generative AI through Andrew Ng's course has been eye-opening. It dispelled myths, clarified misconceptions, and instilled a sense of responsibility regarding the ethical implications of this powerful technology.